Unlocking the Power of Image Datasets for Classification
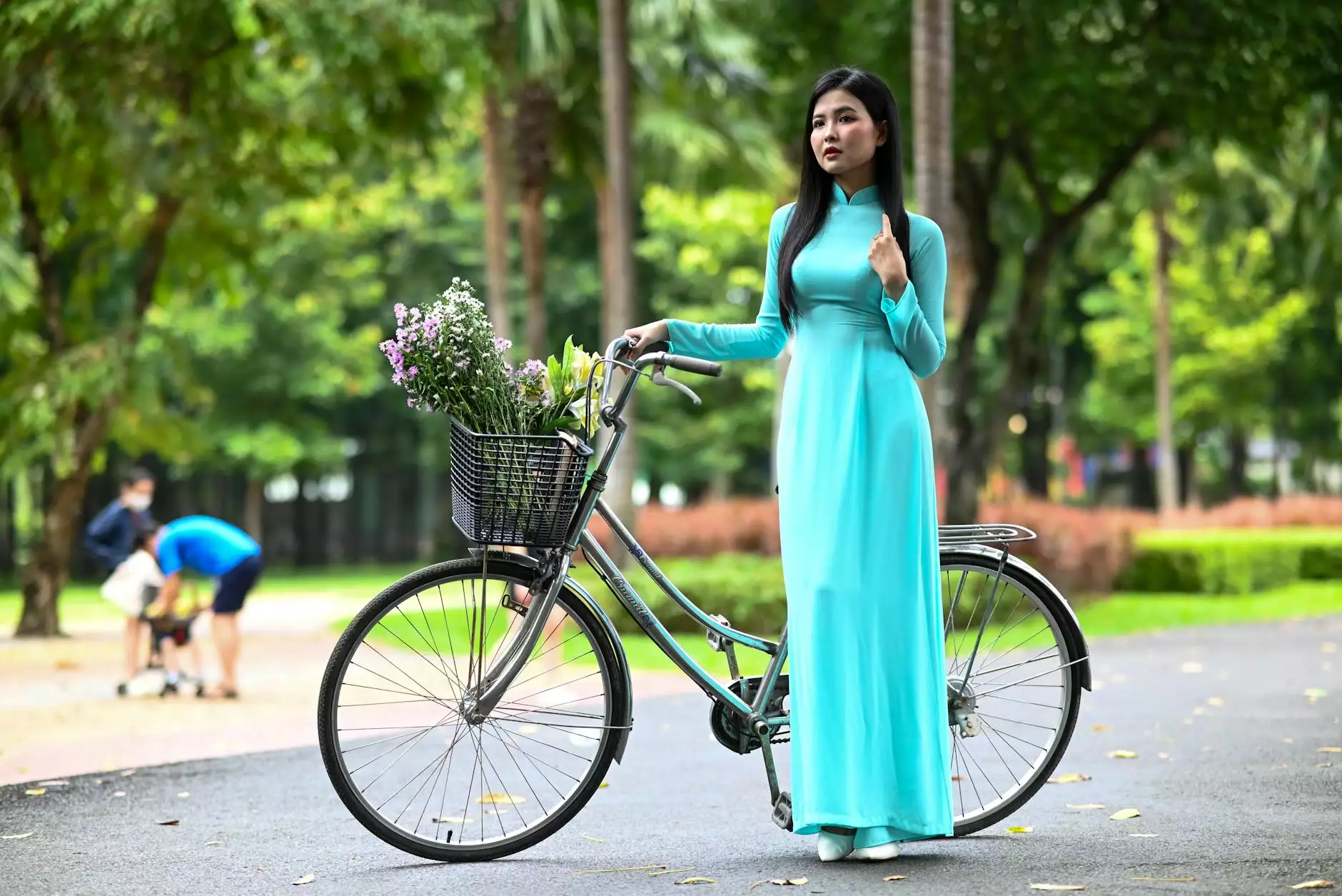
In today’s rapidly evolving technological landscape, the importance of image datasets for classification cannot be overstated. The realm of artificial intelligence (AI) has seen remarkable advancements, particularly in the area of image recognition and processing. At the heart of these innovations lies the concept of data annotation, where precision and quality are paramount. For businesses looking to thrive in the digital age, understanding and utilizing high-quality image datasets is not just beneficial; it is essential.
Understanding Image Datasets
Before delving deeper into the specifics of image datasets for classification, it's crucial to define what an image dataset is. An image dataset comprises a collection of images that are systematically organized for use in training machine learning models. These datasets allow algorithms to learn patterns and features within images, enabling them to make accurate predictions or classifications.
The Role of Data Annotation in Image Classification
Data annotation is the process of labeling data, which in the case of images, involves identifying objects, actions, or features within the visual content. This process is foundational in creating reliable image datasets for classification because labeled data allows machine learning models to understand the context of each image. Here are key components of effective data annotation:
- Object Detection: Identifying and localizing objects within an image by drawing bounding boxes.
- Semantic Segmentation: Classifying each pixel in an image according to its category.
- Instance Segmentation: Similar to semantic segmentation but differentiates between different instances of the same object.
- Image Classification: Assigning a label to an entire image based on its content.
These components are pivotal for generating comprehensive datasets that improve the accuracy of classification tasks across various applications.
The Importance of Quality in Image Datasets
High-quality image datasets are critical for successful machine learning outcomes. A few aspects showcase the significance of quality in these datasets:
1. Enhanced Model Accuracy
Quality datasets lead to improved model training. When the training data is diverse and well-annotated, the resulting machine learning model has a better chance of generalizing well in real-world applications. This is particularly important for applications in industries like healthcare, automotive, and e-commerce, where classification accuracy can significantly impact decision-making.
2. Reducing Bias
Using diverse datasets helps mitigate bias in AI models. A balanced dataset ensures that the model performs consistently across different demographic groups and scenarios. This is especially critical in fields like facial recognition and healthcare diagnostics, where biased datasets can lead to unfair outcomes.
3. Scalability and Flexibility
High-quality datasets support scalability. As businesses evolve, they often need to expand their data requirements. Utilizing scalable annotation platforms like KeyLabs.ai ensures that businesses can efficiently manage large volumes of image data without compromising quality.
Key Labs AI: Pioneering Data Annotation Solutions
KeyLabs.ai is at the forefront of innovation in the data annotation landscape. With a commitment to excellence, KeyLabs provides robust solutions to enhance the creation and management of image datasets for classification. Here’s how KeyLabs.ai stands out:
Comprehensive Data Annotation Tools
The platform offers a wide range of data annotation tools tailored for various image classification tasks. Whether it's for object detection or semantic segmentation, KeyLabs.ai provides intuitive tools that streamline the annotation process, ensuring accuracy and efficiency.
Optimized Annotation Processes
Utilizing advanced algorithms and human expertise, KeyLabs.ai optimizes the annotation workflow to reduce the time and cost associated with data labeling. This efficiency allows businesses to bring their AI models to market faster, providing them with a competitive edge.
Flexible Integration Capabilities
KeyLabs.ai supports seamless integration with existing systems, making it easier for organizations to implement data annotation solutions without overhauling their operational frameworks. This flexibility is crucial for businesses looking to enhance their AI capabilities while minimizing disruption.
Best Practices for Creating Image Datasets for Classification
To maximize the potential of image datasets for classification, businesses should adhere to several best practices:
1. Define Clear Objectives
Understanding the end goal of the image classification project helps determine the types of images that need to be collected and annotated. Objectives can range from product categorization to wildlife monitoring, each requiring specific types of image data.
2. Ensure Diverse and Representative Samples
A diverse dataset encompasses various scenarios and demographics to enhance the model's ability to generalize. This might include images from different angles, lighting conditions, and backgrounds to cover all possible use cases.
3. Quality Over Quantity
While having a large dataset is beneficial, the quality of the images is paramount. High-resolution images with clear annotations are far more valuable than a larger number of poorly annotated images. Clean datasets enhance learning and reduce noise in the model.
4. Continuous Evaluation and Updates
The landscape of image classification is continually evolving. Regularly updating and refining datasets is essential to maintaining model accuracy and relevance. Businesses should incorporate feedback and new data to enhance their classification capabilities.
Future Trends in Image Classification
As technology continues to advance, several trends are emerging in the field of image classification:
1. Automated Annotation Tools
With AI becoming increasingly capable, automated annotation tools are on the rise. These tools can assist human annotators by quickly labeling basic features, thus speeding up the data preparation process.
2. Real-Time Data Annotation
As machine learning models require access to real-time data, the demand for instantaneous data annotation solutions is likely to grow. This trend will allow for quicker responses to changing data environments, particularly in sectors like security and autonomous driving.
3. Enhanced Collaboration in Annotation
The future will likely see a move towards collaborative platforms where multiple users can work together on annihilation tasks, leveraging collective expertise to produce better outcomes in data quality.
Conclusion
The significance of image datasets for classification is profound in driving advancements in machine learning and artificial intelligence. Businesses that recognize this potential and invest in high-quality data annotation processes, such as those offered by KeyLabs.ai, will position themselves at the forefront of innovation. By understanding the critical aspects of data collection, annotation best practices, and future trends in the industry, organizations can harness the power of image datasets to achieve exceptional outcomes in their AI initiatives.
Your Next Steps with KeyLabs.ai
As you venture into the world of image classification, consider leveraging the expertise of KeyLabs.ai. Their cutting-edge data annotation platform not only ensures the creation of high-quality datasets but also supports your journey towards mastering machine learning capabilities. With a comprehensive understanding of the critical factors at play, you have the opportunity to elevate your business and stay competitive in this data-driven era.